Last modified: Friday, October 15, 2010
Eat safer: Novel technology detects unknown food pathogens
FOR IMMEDIATE RELEASE
Oct. 15, 2010
INDIANAPOLIS -- Technologies for rapid detection of bacterial pathogens are crucial to maintaining a secure food supply.
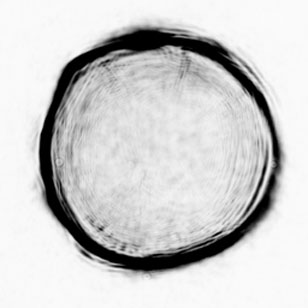
Image courtesy of IUPUI School of Science
'Salmonella Typhimurium'
Researchers from the School of Science at Indiana University-Purdue University Indianapolis (IUPUI) and the Bindley Bioscience Center at Purdue University have developed a novel approach to automated detection and classification of harmful bacteria in food. The investigators have designed and implemented a sophisticated statistical approach that allows computers to improve their ability to detect the presence of bacterial contamination in tested samples. These formulas propel machine-learning, enabling the identification of known and unknown classes of food pathogens.
The study appears in the October issue of the journal Statistical Analysis and Data Mining.
"The sheer number of existing bacterial pathogens and their high mutation rate makes it extremely difficult to automate their detection," said M. Murat Dundar, assistant professor of computer science in the School of Science at IUPUI and the university's principal investigator of the study. "There are thousands of different bacteria subtypes and you can't collect enough subsets to add to a computer's memory so it can identify them when it sees them in the future. Unless we enable our equipment to modify detection and identification based on what it has already seen, we may miss discovering isolated or even major outbreaks."
To detect and identify colonies of pathogens such as listeria, staphylococcus, salmonella, vibrio and E. coli based on the optical properties of their colonies, the researchers used a prototype laser scanner, developed by Purdue University researchers. Without the new enhanced machine-learning approach, the light-scattering sensor used for classification of bacteria is unable to detect classes of pathogens not explicitly programmed into the system's identification procedure.
"We are very excited because this new machine-learning approach is a major step towards a fully automated identification of known and emerging pathogens in real time, hopefully circumventing full-blown, food-borne illness outbreaks in the near future. Ultimately we would like to see this deployed to tens of centers as part of a national bio-warning system," said Dundar.
"Our work is not based on any particular property of light scattering detection and therefore it can potentially be applied to other label-free techniques for classification of pathogenic bacteria, such as various forms of vibrational spectroscopy," added Bartek Rajwa, the Purdue principal investigator of the study.
Dundar and his colleagues believe this methodology can be expanded to the analysis of blood and other biological samples as well.
This study was supported by a grant from the National Institute of Allergy and Infectious Diseases.
Co-authors of "A Machine-Learning Approach to Detecting Unknown Bacterial Serovars" study in addition to Dundar and Rajwa are Ferit Akova, a graduate student at the School of Science at IUPUI, and Purdue University researchers V. Jo Davisson, E. Daniel Hirleman, Arun K. Bhunia, and J. Paul Robinson.