Last modified: Monday, October 18, 2010
Analyzing almost 10 million tweets, research finds public mood can predict Dow days in advance
Bollen to discuss findings on CNBC's "Squawk On The Street" business show Tuesday (Oct. 19) at 9 a.m. ET
FOR IMMEDIATE RELEASE
Oct. 18, 2010
BLOOMINGTON, Ind. -- Measurements of the collective public mood derived from millions of tweets can predict the rise and fall of the Dow Jones Industrial Average up to a week in advance with an accuracy approaching 90 percent, Indiana University information scientists have found.

IU Associate Professor of Informatics Johan Bollen
Researchers at IU Bloomington's School of Informatics and Computing found the correlation between the value of the Dow Jones Industrial Average (DJIA) and public sentiment after analyzing more than 9.8 million tweets from 2.7 million users during 10 months in 2008.
Using two mood-tracking tools to analyze the text content of the large-scale collection of Twitter feeds, Associate Professor Johan Bollen and Ph.D. candidate Huina Mao were able to measure variations in public mood and then compare them to closing stock market values.
One tool, OpinionFinder, analyzed the tweets to provide a positive or negative daily time series of public mood. The second tool, Google-Profile of Mood States (GPOMS), measured the mood of tweets in six dimensions: calm, alert, sure, vital, kind, and happy. Together, the two tools provided the researchers with seven public mood time series that could then be set against a similar daily time series of Dow Jones closing values.
The researchers then correlated the two sets of values -- Dow Jones and public mood -- and used a self-organizing network model to test a hypothesis that predicting stock market closing values could be improved by including public mood measurements.
"We were not interested in proposing an optimal Dow Jones prediction model, but rather to assess the effects of including public mood information on the accuracy of the baseline prediction model," Bollen said. "What we found was an accuracy of 87.6 percent in predicting the daily up and down changes in the closing values of the Dow Jones Industrial Average."
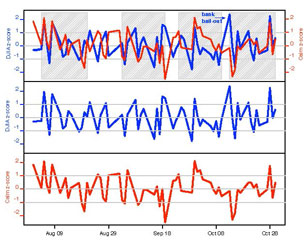
A graph of Dow Jones Industrial Average values (center, blue) and tweets identified with a "calm" mood during a time series (bottom, red) running three days prior are overlaid in the top graph to show gray areas of significant overlap.
By implementing a prediction model called a Self-Organizing Fuzzy Neural Network (SOFFNN) similar to one already used to successfully forecast electrical load needs, the researchers were able to demonstrate that public mood had the ability to significantly improve the accuracy of the most basic models currently in use to predict Dow Jones closing values. Bollen described this particular SOFFNN as a five-layer hybrid neural network with the ability to self-organize its own neurons during a learning process that included information of past Dow Jones and public mood time series values.
"Given the performance increase for a relatively basic model such as the SOFNN, we are hopeful to find equal or better improvements for more sophisticated market models that may in fact include other information derived from news sources and a variety of relevant economic indicators," he said.
The researchers found the OpinionFinder positive/negative sentiment input had no effect on prediction accuracy, while the Calm and the Calm-Happy combination of the GPOMS had the highest prediction accuracy.
"In fact, the calmness index appears to be a good predictor of whether the Dow Jones Industrial Average goes up or down between two and six days later," Bollen said.
The odds of the prediction accuracy rate of 87.6 percent being sheer chance were then calculated for a random period of 20 days and determined to be just 3.4 percent.
Bollen posted the National Science Foundation-funded research paper, available for download here, to the open access science archive arXiv over the weekend of Oct. 16 and Google returned nearly 70,000 hits by Monday (Oct. 18). Joining Bollen and Mao on the paper was Xiao-Jun Zeng of the University of Manchester (United Kingdom) School of Computer Science.
To speak with Bollen or Mao, please contact Steve Chaplin, Indiana University Communications, at 812-856-1896 or stjchap@indiana.edu.